Solving Wordle with Excel
If you're reading this, I assume that you're already familiar with the new online word game Wordle, which was invented by Josh Wardle, and is currently (April 2022) owned and published by the New York Times.
In this game, you have 6 chances to guess a secret 5-letter word, and you get feedback on each guess to help you narrow down your search. I thought it would be fun to build an Excel workbook that can play the game and solve Wordle puzzles automatically.
Design and Data Entry
The first task was to fashion a reasonable look-a-like representation of the grid…

This is built as two Excel tables (the column headers are in a hidden row), the left one to enter letters, and the right one to enter marks.
Each table uses data validation to control what can be entered. The letter table uses an A-Z picklist, and the marking table allows entry of 0, 1, or 2 to indicate the appropriate mark (0=grey, 1=yellow, 2=green). Conditional formatting is used to colour the cell backgrounds according to this number in the marking table (using the same palette as the NYT website) and by making the font colour the same as the background colour, you can’t see the numbers.
Now if our software is going to guess words, it needs to know all the admissible words. It turns out that there are only 2309 of these, and a quick Google search will quickly find a full list. Here’s one place I saw them… https://www.wordunscrambler.net/word-list/wordle-word-list.
I downloaded such a list and put all of the words into a table.
Marking an Answer
The next task is to be able to mark a guess against a secret word. This will need to be written in software. Whilst it may not be obvious yet, if you’re going to be able to judge whether a guess is a good one, you need to be able to ‘test mark’ it against candidate secret words, so let’s consider how to do this.
As you’ll probably know, green is awarded for a correct letter in the right place, and yellow for a correct letter in the wrong place. But there are subtleties – if your guess contains double letters and the secret word contains only one, you’ll only get the ‘better scoring’ one marked. And if the secret word contains a double letter but your guess contains just one, then again you get the better scoring mark.
The way I approached this was to consider that one mark is potentially available from each letter of the secret word, and given to at most one place in the guess. So I loop over each secret letter. If the guess has the same letter in the same place – it gets a green. If (and only if) it doesn’t, then I search the other letters of the guess to see if I can give away one yellow, but ONLY if it isn’t already green (by some other route).
Making a Guess
This is the hard part (of course!).
Let’s consider that we’re part-way through a game. The first thing we do is identify all secret words that are still possible. To do this, we create a list of all 2309 wordle words from our list and mark all the guesses so far against each of these. If it matches the markings so far, we keep it, if not, we remove it from the list.
Now we have a list of candidate secret words. Picking the next guess at random from this list of candidates might work, but it’s not the best possible strategy. A good player will want to squeeze as much information as possible out of every turn. How do we do that exactly?
Well, one approach is to mark every possible guess against each candidate solution in turn and count how many times each possible score comes back. That sounds like a lot of work, but it only takes a few seconds in software if you do it efficiently.
For example, let’s say after 2 guesses, we have this position.

It turns out that there are only 10 possible candidate solutions that it could be…
BILLY, DILLY, DIMLY, FILLY, FILMY, HILLY, IDYLL, IMPLY, MILKY, WILLY
If we were to try an example guess ABACK against each of these possibilities, we’d get a distribution of marks that looks like this…
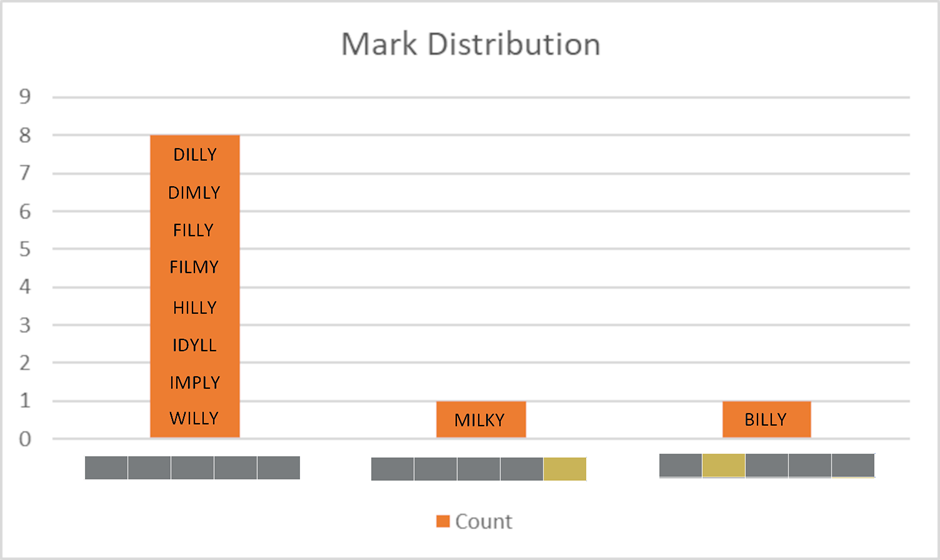
Mark Distribution for ABACK
8/10 (80%) of these words have no letters in common, and would return 5 grey squares, MILKY would award 1 yellow square for the letter K, and BILLY would award 1 yellow square for the letter B.
So 20% of the time (i.e. if the secret word was MILKY or BILLY) we’d have only one possible candidate left after guessing ABACK, but 80% of the time we’d still be left with 8 candidates, which isn’t much of an improvement from the 10 we had before, so most of the time, ABACK wouldn’t be a great guess at this point.
Hopefully, it will be clear that it’s much more useful if we can find a guess that spreads the marks out more - ideally if we can find a guess that would give a different mark for each of the remaining candidates…

An "Ideal" Mark Distribution
Such a guess would guarantee that whatever response we get, we are GUARANTEED to know what the right answer is next time, as whatever the score, it relates to just one possible candidate word.
Unfortunately, in this situation (and most of the time) no such word exists, so we need to pick the best answer from the available choices. It turns out that EMBED is a good guess, as it produces the following distribution of marks.
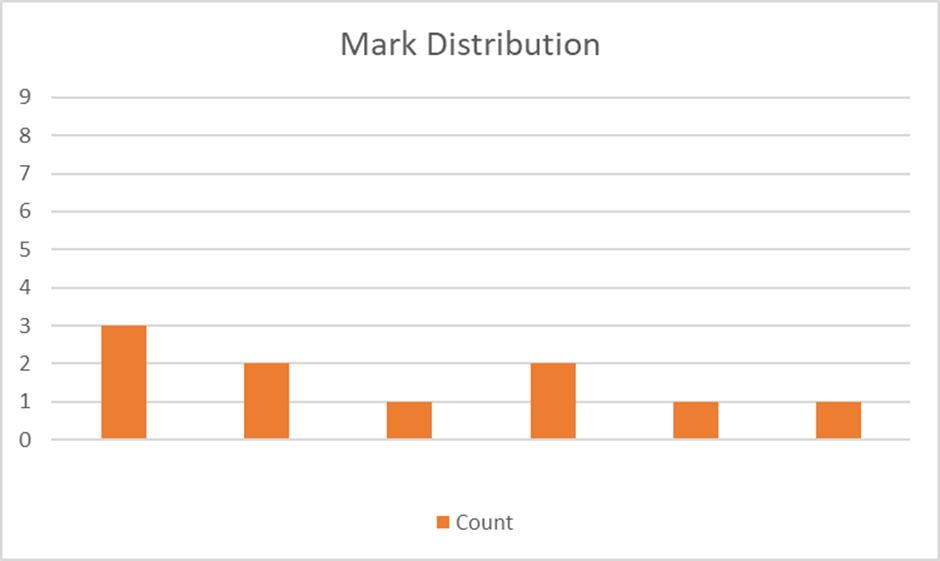
Mark Distribution for EMBED
Here, 30% of the time we will get a unique answer, 40% of the time we will have a choice of 2, and 30% of the time we will have a choice of 3. So the best case happens 30% of the time, and even in the worst case, we’re only left with 3 to pick from. EMBED is clearly a much better guess than ABACK.
How did we find EMBED? Well, it’s the choice with the shortest, tallest bar of all the possible wordle guesses. Put another way, I’ve scored each and every one of the possible guesses (all 2309) using the height of their tallest bar (e.g. 3 for EMBED, and 8 for ABACK) and I’ve picked a guess that has the lowest score. It’s the least worst-case option. Yes, there are probably lots of guesses that are just as good as EMBED. It's not likely to be unique.
If you’re interested, this approach was described in a recent Numberphile video on Youtube, using the game of MasterMind as a simpler example. https://www.youtube.com/watch?v=FR_71HyBytE
Another Approach
It’s not the only way to choose a solution. Statisticians and data scientists might describe these distributions more precisely in terms of their Entropy. Entropy is (sort of) a measure of how random or likely something is.
The distribution we saw under ABACK with 8 of the answers having the same mark (out of more than 200 possible marks) seems pretty unlikely to arise by random chance, so it’s known as a low entropy arrangement. Whereas finding an arrangement where the answers are more evenly spread across lots of different values would seem to be a more likely situation – so EMBED has a higher entropy distribution than ABACK.
There’s a formula to calculate this of course, it’s…

Where pi is the probability of each outcome, and Σ means adding up all the terms within the brackets.
Note that VBA doesn’t have a log (base 2) function, it only offers natural logarithms. But it's simple to convert from a logarithm to another base, as the base change rule is…

loge(2) is a constant (0.693), so to calculate we just need to divide by 0.693. But as we only want to know which word has the largest entropy, this isn’t absolutely necessary, and you could get away with natural logs if you prefer.
In the case of ABACK then, we have an 80% chance of the first option, a 10% chance of the second, and a 10% chance of the third, so the entropy is…

Whereas with EMBED, the entropy calculated by the same formula is 2.45 – indicating a more randomly spread, and therefore more desirable guess. In this application, we want to pick the highest entropy guess.
In practice, the highest entropy choice of all 2309 words is FIELD with an entropy of 2.65. If we examine the distribution of marks we get with FIELD we can see that they’re now spread over 7 different values and that 50% of the time we would get a unique answer. This is even better than we achieved with EMBED, even though both EMBED and FIELD have the same height tallest column.

Mark Distribution for FIELD
So it can be seen that entropy makes more discriminating choices (that should be slightly better), at the expense of a more complicated evaluation function. But in practice, the improvement (measured in how many steps it takes to solve random Wordle puzzles) is very small at best. In trials of about 1000 runs this algorithm (with a step or two yet to be described) averages about 3.65 guesses with the first algorithm and about 3.55 guesses with entropy. It’s a difference of only 2%.
This approach is described further in a 3Brown1Blue video on Youtube: https://www.youtube.com/watch?v=v68zYyaEmEA
Making the final guess
Thus far, I have only described how to choose guesses that efficiently reduce the number of candidate solutions. If this is all you do, you won’t actually solve the puzzle, you’ll just get stuck in an endless cycle of making equally bland and ineffective guesses that fail to reduce the number of candidates to less than 1.
There comes a time when it’s necessary to bite the bullet and actually guess the answer from one of the candidates. Here are my thoughts about when to do that:
- When there’s only 1 candidate solution left.
- It’s REQUIRED to guess this solution at this time. This is a ‘no brainer’
- When there are only 2 candidate solutions left.
- A random guess from these gives you a 50% chance of being right the first time, and a guarantee of being right the second time, so on average it will take 1.5 turns. But if you try one more reduction step, then it will always take 2 turns.
- So it’s better to make a random guess when you have just 2 candidates
- When there are only 3 candidate solutions left.
- A random guess of a candidate gives you a 1/3 chance of being right each time you try. On average it will take 2 turns, or fewer if there’s a candidate that itself scores (1,1,1).
- If your best scoring non-candidate is (1,1,1) (this is quite likely) it will take 2 turns if you reduce one more time, but if your best scoring option is only (2,1) it will take an average of 2.33 turns.
- So a simple rule here might be to always pick the best scoring candidate – on average this is at least as good as any other strategy. However, if you’re on your 5th turn, you might prefer the certainty of taking that (1,1,1) option. But if you’re on your 6th turn you might prefer to take the 1/3 chance you might get it right, rather than the certainty of failure. It’s complicated and context-sensitive.
- I’ve not analysed the situation with 4 or more candidates, but it’s only going to get more complicated. So I’m simply going to assume that if the case with 3 candidates is marginal, then with 4 candidates any benefit of making a guess from the candidates will mostly be lost. So in my code, if I have 4 or more candidates - I reduce, unless I’m on my last guess.
Here’s a very quick and easy way of implementing the above strategy. If you have 3 candidates or fewer (or you are on your last guess) only pick guesses from the candidates, not the whole set of 2309 wordle words.
Performance Optimisations
Marking and scoring 2309 words against a shortlist of candidates during the mid-game isn’t too onerous, but for the first and second guesses, it does take tens of seconds. Here I can recommend two important optimizations.
The first guess is always from the same “no information” starting point. So it makes sense to score all 2309 words just once and to store all the scores (for future reference) along with each word. You could then ALWAYS start with the best word if you really wanted to, but that’s not very interesting. A more varied approach would be to pick something at random from the top 5% or 10% best-scoring words.
The second guess typically also has a lot of candidates to look at. But you’re still just narrowing down the possibilities, you don’t need to examine all possible guesses. It would be astonishing if you can’t find an excellent choice amongst (say) 250 examples that wouldn’t be 99% as good as searching through the whole set of 2309. So yes, in the second round, I only examine 10% of all possible guesses. I only look at the full set from the 3rd round.
Thirdly, when dealing with markings, and comparing to see whether a test word matches the mark of a marked word, it’s not efficient to compare each cell in turn. Instead, I store and compare the marks as a 5 digit ternary (base 3) number. So instead of 5 comparisons, I perform one.
And finally, a surprising effect of the 3 candidates or fewer trick to pick a guess from the candidates, rather than from the whole dictionary, made much more difference to the speed of execution than it did for the average number of guesses. In practice, instead of taking about 5 seconds to solve a game, it takes about 2.5 seconds.
Going for Broke
The success of Wordle has inspired some 'more difficult' variants on the theme.
Quordle https://www.quordle.com where the goal is to solve 4 puzzles at once in 8 turns.
Octordle https://octordle.com/ where the goal is to solve 8 puzzles at once in 13 turns
and even Absurdle https://qntm.org/absurdle which is Wordle, with the secret word changing dynamically as you play, trying to cut you as little slack as possible.
It's perfectly possible to extend the Excel workbook and the code to an 8 puzzle variant, and here it is (with marking tables hidden)
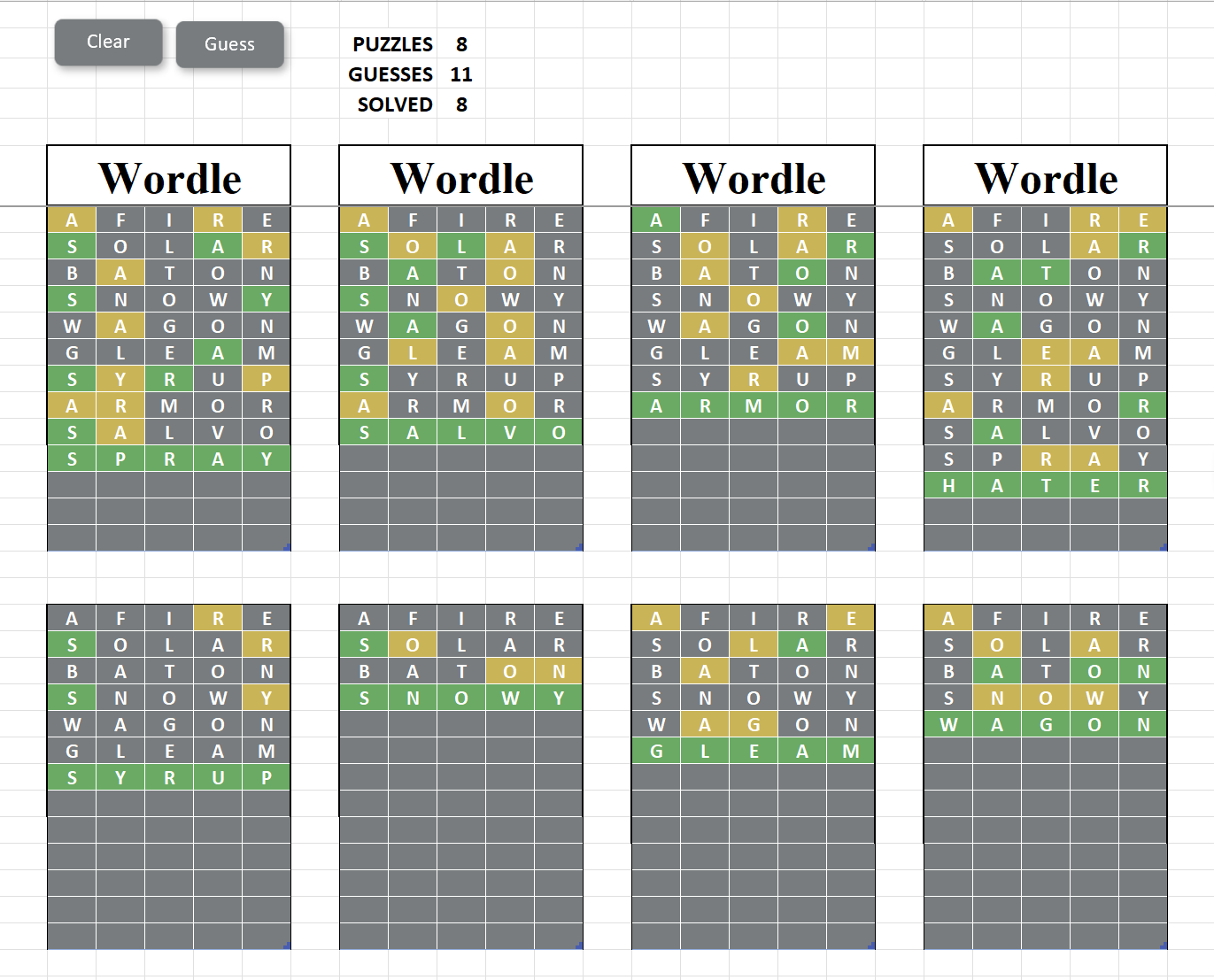
Extension of Wordle solver to crack up to 8 puzzles at once
Apart from the obvious need to work with 1, 4 or 8 tables (as selected by the PUZZLES value) the extensions to the algorithm are not all that major.
The Making a Guess routine produces a single, merged list of all candidate solutions for all puzzles before searching for the largest entropy guess that would distribute them (as before). The logic that argued when to cut over to guessing the answer rather than reducing the candidates is applied only to this merged list (as it's only really justified when solving the final puzzle) so an additional check is made that if (at any time) there is only one candidate left for a puzzle then that candidate IS the next guess, ignoring everything else.
The typical performance of this algorithm is about 11 to 11.5 guesses to solve the whole puzzle, taking about 30 seconds a time.
-oOo-